In the pet food industry, business decisions about product advancement are based on comparative palatability assessments using data. Pet preferences are most often determined using two-bowl trials. Data from these or any comparisons must be carefully analyzed to tell an accurate story. The first step in this analysis is to...
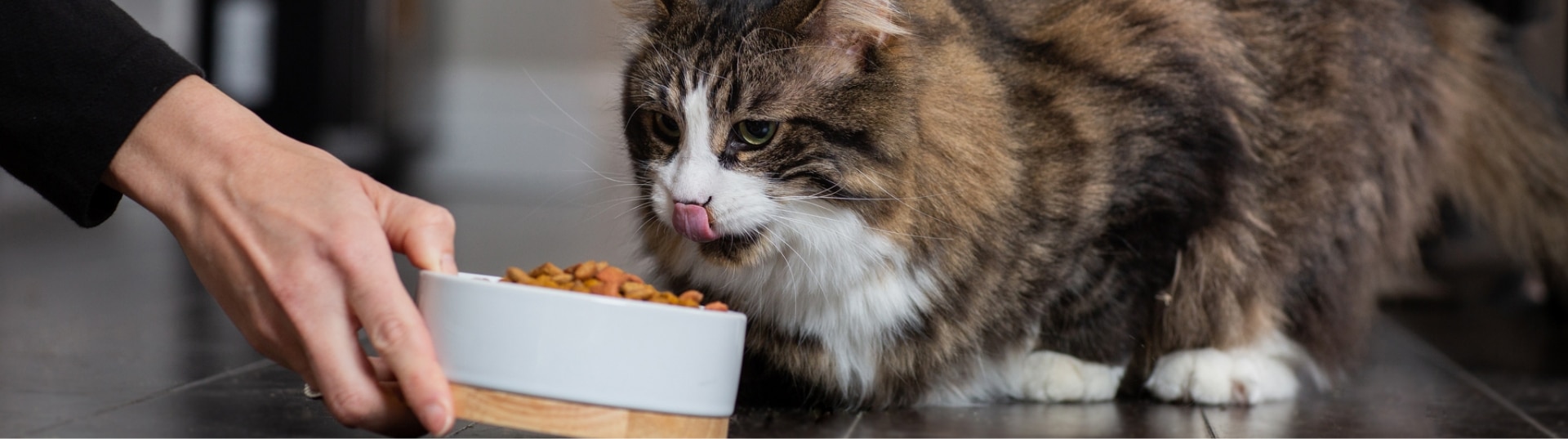
Data-Driven Innovation for Robust Product Consistency
Palatants are flavor enhancers used in pet foods to improve their palatability and increase pet acceptance. Developing an effective palatant involves a systematic approach to selecting the best combination of ingredients that provide the optimal aroma and taste for cats and dogs. However, some ingredients have inherent natural variability that must be managed to ensure the robustness and consistency of the palatants.
To address this, an innovative data-driven approach utilizing several analytical techniques can be implemented. Chemical characterization of raw materials—focusing on parameters such as protein, ash, fat, moisture, amino acids, and biogenic amines—is essential for monitoring variability between different types and suppliers of these raw materials.
Ensuring robustness and consistency involves several key steps:
1. Comprehensive Ingredient Analysis: Regularly analyzing raw materials for their chemical composition helps identify and control variability. This analysis includes measuring levels of proteins, fats, moisture, and other critical components.
2. Predictive Modeling: Utilizing advanced predictive modeling techniques can classify chemical fingerprints of raw materials quickly and accurately. These models help in anticipating and compensating for variations, maintaining a consistent quality in the final product.
3. Supplier Evaluation: Continuously evaluating suppliers based on the chemical profiles of their materials ensures that only the most consistent and high-quality raw materials are used.
4. Quality Control Protocols: Implementing stringent quality control protocols at various stages of production helps detect and address deviations from the desired chemical profiles.
By integrating these strategies, the development of palatants can achieve higher robustness and consistency, leading to better product performance and increased pet satisfaction.
DATA-DRIVEN ANALYTICAL TECHNIQUES
These techniques involve leveraging data analysis methods to inform decision-making.
PREDICTIVE MODELING FOR CONSISTENCY
The integration of fingerprint data with advanced predictive modeling techniques allows for the classification and comparison of raw materials. Machine learning algorithms can be trained on the chemical fingerprints to predict the quality and consistency of those samples, ensuring that variations are detected and managed effectively.
IMPLEMENTATION STRATEGY
This step is important to develop actionable strategies for optimizing processes and outcomes.
CONCLUSION
A data-driven approach to managing the variability of natural raw materials in palatants and pet food can significantly enhance product consistency and robustness. By leveraging advanced techniques such as chemical characterization, fingerprint analysis, and predictive modeling, we ensure our products meet the highest standards of quality and palatability for pets.
This innovative strategy not only maintains consistency but also drives continuous improvement in our product offerings. By comprehensively analyzing raw materials, rapidly classifying chemical fingerprints, and implementing predictive models, we can anticipate and mitigate variations, leading to a more reliable product.
Moreover, continuous supplier evaluation and stringent quality control protocols ensure that only the best ingredients are used, further reinforcing the robustness of our palatants. This holistic approach not only meets current quality standards but also sets a foundation for ongoing enhancement and innovation in pet food flavor enhancers.
For more information, contact AFB’s Customer Technical Insights team by visiting afbinternational.com/contact or your AFB Account Representative.